When on March 13 2020 the number of Covid-19 cases and deaths in Europe exceeded the rest of the world (excluding China) for the first time, the World Health Organization declared Europe as the new epicenter of a worldwide spreading disease it had only declared a pandemic two days earlier. Five days later, the Guardian reported that 250 million people all across Europe were in lockdown. However, the severity of the health measures that different governments throughout Europe enacted varied quite dramatically. Italy, which was hit especially hard early on, had already put local municipalities in its Northern regions under strict quarantine on February 22, and had closed schools and universities nationwide on March 4. The UK government, on the other hand, had not enacted any social distancing guidelines prior to March 18, but then moved to more stringent recommendations on March 23. (Only four days later, Prime Minister Boris Johnson announced that he had tested positive for Covid-19.)
In this situation, Sweden chose a unique path, in that it mostly remained “open for business,” enforcing only fairly minimal restrictions and trusting instead that citizens behave responsibly. Optimists hoped that this would be enough to prevent Covid-19 from getting out of control, while pessimists feared enormous death counts when people go about their lives as if nothing was amiss.
Now, four months later, it appears that neither the optimists nor pessimists were right: Swedish mortality rates fall right between those of countries that acted swiftly and drastically and countries that acted later and not as decisively. But while it might seem “obvious” to the casual observer that the answer “must” lie between the extremes, we can learn a lot about how this actually came about in detail. This is the subject of a recent fascinating paper by Lynn Kamerlin and Peter Kasson, published in the Journal Clinical Infectious Diseases.
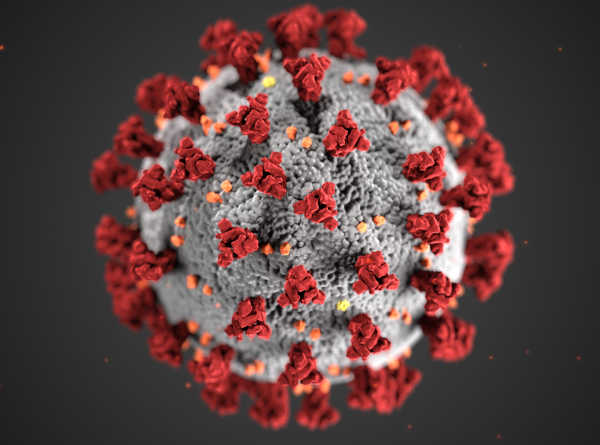
Image credit: CDC
The most common approaches for scientifically describing the spread of an epidemic, amply discussed over the past months even in the general press, are so-called “compartmental models”. These simply count the number of people in a population who are sick on any given day, and from that alone try to predict how many of the healthy people will catch the disease on the next day. One can add bells and whistles to such models, such as recovery with lasting or limited immunity, finite incubation times, or asymptomatic patients. But the key underlying approximation of nebulously spread out sick people somehow infecting healthy people always stays. These models make no attempt to describe how individual sick people would actually encounter healthy ones and infect them; they instead just think in terms of an aggregate probability for spreading the disease, quantified by an empirical parameter called R0 (“R-naught”). The enormous advantage of this simplification is that these models can be solved almost by paper and pencil. But the fact that the entire underlying dynamics for how the disease actually spreads through a population is summarily packaged into a single empirical parameter comes at a price: there are many crucial public policy questions which such models simply cannot answer. What would happen if we close schools? Would it help if people only go to work two days a week, and if yes, how much? Are small villages equally at risk as big cities?
To examine such questions, Kamerlin and Kasson decided to model the spread of the disease with much finer granularity, using something that is called “individual based modeling”. Of course, one cannot attempt to replicate the lives of about 10 million Swedes on a, say, hourly basis in a computer. But one can account for geographic and demographic data: population density, household size, age distribution, employment rates, different age levels for schools and workplaces, etc. Many of the necessary raw data are available, but the model then still contains a number of free parameter (such as: if 15 people share an office and one of them is sick, how many of the remaining healthy ones will catch the disease?), which need to be somehow determined. One way to get hold of these numbers is to adjust them such that they “postdict” a part of the disease dynamics that had already been observed. Kamerlin and Kasson for instanced checked, which values would best describe the development of observed cases and deaths between late March and early April, as predicted from the known “initial condition” of mid March. And if no good way of confining the parameters was available, the authors simply tested a plausible range in order to assess, how strongly the answer depended on these parameters.
A key first result of their study is that if Swedes had taken the absence of governmentally enforced restrictions as a license to ignore the pandemic, then the pessimists would have been right: many people would have died: by June 2020 somewhere between 80,000 and 90,000, almost 1% of the entire Swedish population. In reality, only about 4,500 people had officially died by that point in time, about 20 times less, even though (per capita) still more than other European countries that decided to react more forcefully. Germany for instance had twice the number of deaths at that point, but it also has an eight times larger population.
- Percentage of 100,000 people who would have died if everyone ignored the pandemic 85%
- Percentage of 100,000 people who actually died 4.5%
So why didn’t as many Swedes die as was feared? Kamerlin and Kasson argue that even as the government didn’t force people into lockdown, many Swedes did so anyways: voluntarily. And as the Swedes decided in large numbers to self-isolate, work from home, socially distance themselves from neighbors and friends, and cut down on travel, the case count drastically lagged behind the worst case scenario of a population that simply ignores the pandemic. Even better measures could have been achieved—and were in fact achieved in other countries—by more drastic interventions. Would this have been worthwhile? Many people currently point out that extensive lockdowns hurt the economy, and one has to balance concerns for the health of citizens with the health of the economy, because a depression also leads to long term suffering and death. However the situation is neither that binary, nor in fact that simple: if many people get sick and die, that will negatively impact the economy as well. In fact, for the case of the Spanish Flu it has been argued that a stronger pandemic response yielded a better economic recovery, in places where it was enacted. And early on during the Covid-19 pandemic, economist Luigi Zingales had estimated that letting the pandemic run its course in the US would incur costs of 65 trillion dollars, which is a lot more than what a judiciously enacted lockdown would cost.
So a responsible citizenry decided to voluntarily do the right thing, and many tens of thousands of lives were saved as a result. This sounds like a success story, but there are also drops of bitterness: even as the scenario played out much more benignly than the pessimists feared, it was clear that the death count would still be substantial. Care needed to be taken to not overwhelm the healthcare system, because once that happens, many preventable deaths ensue. Sweden had therefore approximately doubled the number of intensive care unit (ICU) beds, and it was found that the ICU capacity was in fact never exceeded. This sounds good on the surface, but looking at the numbers, one also finds that a sizable fraction of deaths occurred outside ICU units. This was especially true for older patients, who were more likely to die than to be admitted to an ICU. Why weren’t these people helped?
There is a neat way to quantify this: divide people in age groups and then ask the following question: if those people tested positive for Covid-19, what were the odds of being admitted to the ICU (call that pICU), and what were the odds of dying from the disease (call that pdeath)? Now look at the risk ratio pICU/pdeath. If this ratio drops below 1, then people are more likely to die of the disease than being admitted to the ICU. The figure below, taken from the paper by Kamerlin and Kasson, shows this risk ratio, for a set of age groups, both for Sweden and for the US. Clearly, in Sweden elderly patients, above the age of 70 to be precise, were more likely to die outside the ICU. Given that ICU capacity in Sweden was actually never overwhelmed, this poses an important question: Why weren’t these people helped?

Risk ratio pICU/pdeath for Covid-19 positive people, divided into age groups, both in Sweden and in the US. The horizontal red line has the value 1. If the risk ratio drops below 1, patients are more likely to die than to be admitted to an ICU. Figure taken from the paper by Kamerlin and Kasson.
Kamerlin and Kasson have a suspicion: the predicted prognosis might have been used as a criterion of admission. In order to prevent the ICU system from being overwhelmed, elderly patients with a poor prognosis might not have been admitted in order to “save” the bed for potential future younger patients with a bigger chance to recover once in the ICU.
This type of “medical triage” poses very difficult ethical questions, which have led many countries and medical associations to draft guidelines intended to support health care professionals in making these decisions, without feeling left alone while being forced to bear the moral burden of having to call the shots on life and death. These guidelines tend to consider medical prognosis as an indispensable part of such decisions, as opposed to a more equitable but overall less effective “first come first serve” approach. Hence, the Swedish decision to factor in prognosis is not in and of itself striking. But then, pointing to the low number of ICU admissions as an indication for success, as recently done by Anders Tegnell, the scientist who devised Sweden’s response to the pandemic, seems disingenuous: people cannot die in the ICU if they are not admitted to the ICU.
Still, there is a positive lesson and a spark of optimism here: social distancing, self-isolation, and a number of other “nonpharmaceutical interventions” clearly help to curb a pandemic—provided people follow them. The case of Sweden has shown that a large fraction of the population may indeed support such measures, even if not expressly required to do so by the government. Of course, the real question is, how transferable this is to other countries—with the obvious elephant in the room being the United States, where cases are presently skyrocketing. This is particularly pressing, given that in “the land of the free” any type of governmental mandate tends to be viewed with great skepticism. Without legal mandates, the obvious alternative is to lead by example. Unfortunately, the present US administration has persistently down-played the seriousness of the situation and dismissed even the simplest measures of guarding against it (such as face masks), both of which significantly reduces the willingness of many citizens to voluntarily do what has clearly helped in the rest of the world. This, however, is not a problem for epidemiologists; it is one for social and political scientists. Let us hope they find an answer soon.
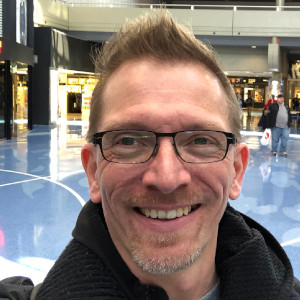
Markus Deserno is a professor in the Department of Physics at Carnegie Mellon University. His field of study is theoretical and computational biophysics, with a focus on lipid membranes.
0 Comments