by Markus Deserno
One of the fundamental facts of statistical physics is that microscopic systems are subject to constant thermal fluctuations. They are fundamentally random, but still exhibit many regularities that permit us to learn a great deal about those systems. For instance, any individual quadratic degree of freedom of the form $\frac{1}{2}Kx^2$ has an expected value of the energy equal to half the thermal energy, or $\frac{1}{2}k_{\rm B}T$. This so-called equipartition theorem in particular implies that we can measure the spring constant $K$, provided we can measure the variance of the fluctuations: $K=k_{\rm B}T/\langle x^2\rangle$.
A well-known application in the field of fluid lipid membranes is that we can determine their bending rigidity from the power spectrum of shape undulations. If we expand a membrane’s shape $h(\boldsymbol{r})$ into a Fourier series, with expansion coefficients $\tilde{h}(\boldsymbol{q})$, then (in the simplest case) the bending rigidity $\kappa$ is given by $\kappa=k_{\rm B}T/[q^4\langle|\tilde{h}(\boldsymbol{q})|^2\rangle]$ (possibly up to some nuisance factors that depend on how we define the Fourier transform). This technique has been widely popular—both in experiment and in computer simulations. Extending it by additional degrees of freedom (say, lipid tilt), for which we also have microscopic models, yields formulas that permit us to extract not just the bending rigidity but also those other moduli appearing in these more sophisticated theories, provided we can measure their associated fluctuations as well.
Until fairly recently the limiting factor for doing this in computational incarnations of “flicker spectroscopy” was the difficulty of getting enough statistics—especially for the long-wavelength modes, which tend to temporally decorrelate very slowly (with a rate somewhere in the $q^3$ or even $q^4$ ballpark). But as with (almost) everything in computational physics, the continuing progress in hardware and algorithmics has rendered this less and less of a problem.
This has led to a new challenge, one which we could mostly ignore until recently, given the poor sampling we had at our disposal. If we can legitimately aim for a statistical uncertainty of, say, 10%, we also must ask: what are the systematic uncertainties?
As with everything connected with the evil enemy called “systematics,” the sources of trouble could reside anywhere. Limits in the theoretical description, finite size effects, artifacts due to the way we define our observables, ambiguities about the correct order to calculate some effect, and much more. In a recent paper my student Muhammed Ergüder and myself have looked at a few of those, and we were surprised to see how big the effects really are. (A more proper description of our mood is probably: sometimes annoyed, sometimes terrified.) But no worries, peace is just a few articles away.
Much of what we did revolves around the question how to even define the kind of lipid membrane which all existing theories talk about. Recall that whether we use a “plain Helfrich description,” or whether we decorate this theory with additional terms (such as tilt or twist, or even breathing modes), the fundamental theoretical construct is a two-dimensional surface, possibly equipped with some additional degrees of freedom, flopping in the thermal breeze (as David Needham is wont to say). But our computer simulations usually do not contain undulating surfaces; they contain lipids. So one of the first steps is to somehow interpolate a surface between these molecules. How should one do that?
Researchers over the years have used various conventions for doing so, and it really didn’t matter all that much during the olden days, when we were lucky to get things accurate within a factor of two. But now it matters. In fact, it turns out that conventions can change the measured value by maybe 50%, and it’s not quite obvious ahead of time which way the deviation goes, and what’s a good or a poor choice. For instance: if we wish to find a representation of the mid-surface of the bilayer, it seems very natural to interpolate this via the tail beads of lipids, which are closest to that surface. This, however, turns out to be a remarkably poor choice. The reason is that the tail ends of lipids have an annoyingly high probability to “snorkel up” to the interfacial region of the membrane, giving rise to highly localized “blips” in the membrane interpolation, which add high-q noise that systematically increases the apparent value of the bending rigidity. (Why “increase”? Because the fit has a tendency to compensate for the high-q increase in the power spectrum with a decrease at low q, which is where we “pick up” the value of the bending rigidity.) It is hence much better to represent the surface of a membrane by interpolating each leaflet via atoms in the head group region (anyone will do, really), and then average these two surfaces. (An even better idea is to use all atoms for the interpolation, as we also show.) Lots of other dependencies of this type exist, some of which end up creating trends we cannot fully explain, but which are clearly visible in the data, for a wide variety of lipids.
Another interesting question revolves around the definition of lipid directors, whose longitudinal and transverse components, after Fourier transforming, give rise to power spectra that afford us a very efficient measurement of bending, tilt, and twist moduli from remarkably small systems—as first demonstrated by Frank Brown. Curiously, it turns out that it depends how precisely one defines these components, though. Does one define a lipid director as a unit vector and then just consider its xy-component? Or does one normalize the z-component of the director to 1 and consider the xy-component of that vector? The latter might look approximate, or at the very least strange, but it so happens that this is exactly what falls out of the mode calculation. So, which one is it? We might fret about this, but then decide to just ignore this entire question because, clearly, the difference between these two methods is of higher order, too unimportant to take seriously in linearized Monge gauge. However, even though naïve “order counting” would agree with our assessment, in practical terms the difference is very much non-negligible—typically about 30%, which is very large! Indeed, Frank Brown has been alerting us all for a number of years now to this important point. We should all listen better!
Besides all kinds of “definition questions,” there are also subtle technical implementation questions one should be careful about. Here’s one example: as mentioned above, undulation modes have an annoyingly large temporal autocorrelation, which forces us to run really long simulations. But it also implies that if we wish to actually assign a statistical uncertainty to a membrane’s power spectrum, we must remember that the amplitudes measured over the course of a trajectory are clearly not statistically independent. Therefore, the error of the mean is not just the standard deviation divided by the square root of the number of samples. There’s a correlation correction that makes the true error larger. This matters for two reasons. First, not having proper error bars for the power spectrum makes it impossible to assess how plausible our fits to it are (say, via the “goodness of fit”). How, then, would we even know our theoretical model works? And second, the autocorrelation time strongly depends on the wave vector: smaller vectors lead to larger autocorrelation times, and hence to larger correction factors for the actual statistical uncertainty. Not accounting for this leads to a q-dependent trend in error underestimation, which does not just affect the precision of the obtained moduli, but could systematically shift them. Of course, there are well-known methods for how to account for these correlations, most notably data blocking. But the point is: we really should use them here!
Our paper discusses quite a number of other subtleties. We were half tempted to call it “Everything you always wanted to know about power spectral analysis (but were afraid to ask)”. That would have been a lie, though. Despite being a formidable chamber of horrors, we clearly have not talked about everything that’s worth mentioning. (After all, for a topic that keeps throwing new complicated details at you, it’s challenging to find the point where you stop doing research and start writing the paper—but at some point you just have to start.) Hence, there’s more work to do, more tests to be performed, and more idiosyncrasies to be understood. Luckily, other groups have also been actively investigating this, and we’re hoping that in the not too distant future we have a much better understanding how to make best use of the much better power spectra which we can now routinely simulate. Given that there are so many fun problems being explored right now (such as mixed membranes, or leaflet asymmetry), it will be important that we can trust our elastic measurements before we dive into exciting and unknown physics.
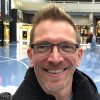
Markus Deserno is a professor in the Department of Physics at Carnegie Mellon University. His field of study is theoretical and computational biophysics, with a focus on lipid membranes.
0 Comments